An Artificial Intelligence System Anticipates How 2 Protein Molecules will Bind Together Instantly
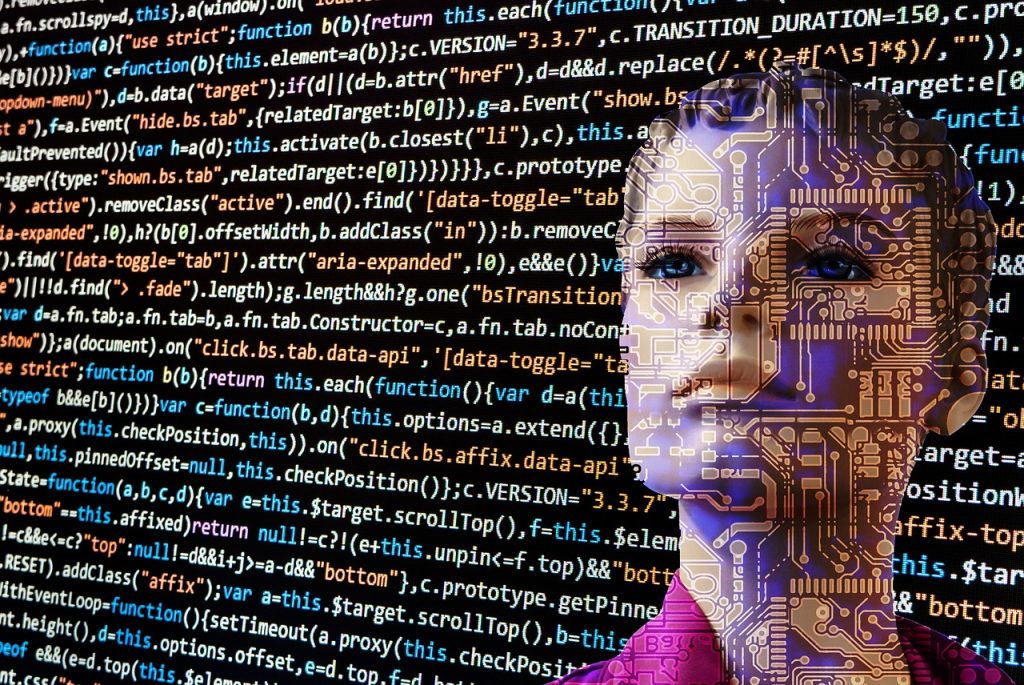
Scientists could use the AI framework to help accelerate the expansion of new medications.
Determinants are small proteins that the resilient structure can attribute to explicit portions of an illness to destroy. One promising armament in the battle against SARS-CoV-2, the illness that produces Covid-19, is a designed offsetting chemical that binds to the infection’s peak proteins and prevents it from reaching a living cell.
Researchers must see just how that link will happen to promote a successfully produced immunizer. Protein with irregular 3D models comprising multiple creases might remain with each other in a great number of mixes, making finding the optimal protein composite amongst literally uncountable candidates extremely difficult.
To level out the contact, MIT researchers developed an AI model for predicting the complication that would emerge when 2 protein molecules bind simultaneously. Their method is in the middle of 80 and 100 times more rapidly than preferred programming methods, and it consistently forecasts protein complexes that are nearby to authentic patterns that have already been provisionally recognized.
This strategy could help researchers to better understand a few natural changes involving protein interaction, such as replication of DNA and mending; it might hurry up the most frequent progression of evolving newfangled drugs.
Protein-protein interaction
Equidock, the approach developed by the researchers, is based on unyielding body cropping, which takes place when 2 proteins confer by revolving or rendering in three-dimensional space but their forms do not match. The framework involves the three-dimensional layouts of 2 protein molecules and translates these to three-dimensional representations that the brain organization can comprehend. Proteins are made up of amino acid chains, and each one of these amino acids has its center in the diagram.
The researchers compiled quantitative data into the database to understand how goods may alter if they were rotated or transformed into three-dimensional space. The framework also includes quantitative information that ensures that proteins usually attach in the same way, irrespective of where they are in three-dimensional space. This is in what way proteins interact with one other in the body.
The AI approach recognizes components of the 2 protein molecules that are expected to work together and form material reactions, termed as restricted pocket emphases, using this information. Then it employs these foci to join the 2 proteins with each other to form a complex.
Maybe the most difficult aspect of creating this approach was incapacitating the deficiency of data preparation. There are so few tests on 3-dimensional knowledge of proteins, it was very imperative to slot in quantitative data into Equidock.